I am pleased to announce that Frederick P. (Fritz) Roth, PhD, known worldwide for his work in computational biology and genomics, will join the School of Medicine as professor and chair of the Department of Computational and Systems Biology (CSB), effective October 1, 2023. Dr. Roth will also lead the development of the school’s institutional computational genomic strategy. Since 2011, Dr. Roth has been jointly appointed as professor of molecular genetics and computer science at the University of Toronto’s Donnelly Centre for Cellular and Biomolecular Research and senior investigator at Sinai Health System’s Lunenfeld-Tanenbaum Research Institute (LTRI), also in Toronto. His research team at both the Donnelly Centre and LTRI carries out computational and experimental genomics research and pursues technology development through fundamental discovery and clinical applications. |

School of Medicine Generates $108M Spike in NIH Funding
From the Senior Vice Chancellor for the Health Sciences:
“I’m excited to share that preliminary data from the National Institutes of Health (NIH) on grants awarded during federal fiscal year 2022 (FFY22) indicate that funding to the University of Pittsburgh School of Medicine has increased to an estimated $550M—a projected rise of nearly $75M over FFY21. This 16% increase far surpasses our challenge goal of achieving 10% growth in FFY22, which ended Sept. 30. Added to the growth of $33M in FFY21, NIH funding to Pitt Med has grown an impressive $108M (24%) over the last two years. These are remarkable accomplishments that directly reflect the extraordinary research excellence and innovation of our Pitt Med faculty. You are to be congratulated.
NIH awards to Pitt Med represented 81% of the overall $675M awarded to the University of Pittsburgh as a whole in FFY22—which itself represents institutional growth of 13% over FFY21. While the finalized NIH 2022 data should be released later this month, the preliminary data move both our School of Medicine and the University from a rank of No. 11 in 2021 to an estimated rank of No. 3 in 2022 for the University and a rank of No. 6 for the School of Medicine.
We are also particularly pleased by the significant increase in the number of large awards. Grants of $10M or more to the University increased from 10 in 2021 to 15 in 2022, with all of these awards coming to the School of Medicine (a $5.6M, 13% year-over-year funding increase). Grants ranging from $5M-$10M grew from 18 to 20, with all 20 of these in the School of Medicine, representing a year-over-year funding increase from $32.8M to $66.9M (104%). Pitt Med also received 25 new grants in the $1M-$5M range (an increase from $199M to $217M or 9% year-over-year).
We have every confidence that our stellar Pitt Med faculty can maintain or even better these NIH award levels in FFY23. We also urge you to continue diversifying your research funding portfolios by considering opportunities from the Department of Defense, the VA, the National Science Foundation, and large philanthropies. Given the vast resources of Pitt Med and UPMC, our greater Pitt campus, and our increasingly engaged communities, we also urge you to continue pursuing opportunities for collaborative and interdisciplinary team science for which we are so expertly poised.
Once again, congratulations on an astounding year of research growth. I look forward to your continued success.”
Ivet Bahar steps down as chair of Computational and Systems Biology
Message from the Dean
Ivet Bahar, PhD, founding chair of the Department of Computational and Systems Biology (CSB), will be resigning from her role effective December, 31, 2022 after 21 years of exceptional service. Ivet also holds the John K. Vries Chair in Computational Biology, has served as associate director of the University of Pittsburgh Drug Discovery Institute since 2010, and was elected to the National Academy of Sciences in 2020.
Ivet earned her MS in chemical engineering from Bogazici University, Istanbul, and her PhD in physical chemistry from Istanbul Technical University. She served for several years as professor of chemical engineering and founding director of the Polymer Research Center at Bogazici University before Pitt Med recruited her in 2001 to create a new Center for Computational Biology and Bioinformatics. The center’s success led to the formation in 2004 of the Department of Computational Biology (later renamed Computational and Systems Biology), one of the first such departments in the nation, with Ivet as founding chair.
In her nearly two decades at the helm of CSB, Ivet has firmly established a program of international impact. CSB’s highly interdisciplinary research faculty of both physical and biological science experts collaborate widely throughout the University, across the nation and around the world. The department also reflects Ivet’s longstanding commitment to developing the next generation of outstanding and diverse scientists in the field. In 2005, she co-founded the internationally acclaimed PhD program in computational biology, offered jointly with Carnegie Mellon University. In the past decade, she also established CSB’s Training and Experimentation in Computational Biology summer program for undergraduate students, funded by the National Science Foundation, and also supported the development of CompBio, one of the eight sites of the UPMC Hillman Cancer Center Academy for high school students. Both summer programs pull heavily from highly diverse academic, economic, and ethnic student populations.
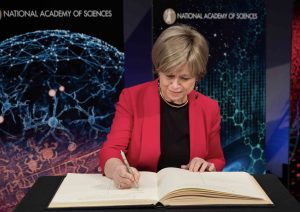
Ivet signs the historical book during the inauguration of her elected membership of the National Academy of Science
Ivet is a pioneer in structural and computational biology, having developed widely-used elastic network models for examining the collective dynamics of proteins. In the past decade, she led the development and use of quantitative molecular and systems pharmacology models and methods, with applications to neurological disorders, cancer biology, and other diseases. More recently, she has contributed to understanding the molecular origin of multi-inflammatory (long) effects of COVID-19.
Ivet will be joining the State University of New York at Stony Brook as director of the Laufer Center for Physical and Quantitative Biology, a move that will also allow her to live closer to her children and grandchildren. Jeremy Berg, PhD, professor of computational and systems biology and associate vice chancellor for science strategy and planning, health sciences, is leading the search committee to find Ivet’s successor and will also serve as interim department chair.
Please join me in thanking Ivet for her vast contributions to our School of Medicine and wishing her the very best in her future.
Anantha Shekhar, MD, PhD
Senior Vice Chancellor for the Health Sciences
John and Gertrude Petersen Dean, School of Medicine
University of Pittsburgh.
Combination of Dynamical Systems Theory and Machine Learning Predicts Cell Fate Governing Equations
![]() Yan Zhang |
![]() Ivet Bahar |
![]() Jianhua Xing |
Single-cell (sc)RNA-seq, together with RNA velocity and metabolic labeling, reveals cellular states and transitions at unprecedented resolution. Fully exploiting these data, however, requires kinetic models capable of unveiling governing regulatory functions. Here, we introduce an analytical framework dynamo (https://github.com/aristoteleo/dynamo-release), which infers absolute RNA velocity, reconstructs continuous vector fields that predict cell fates, employs differential geometry to extract underlying regulations, and ultimately predicts optimal reprogramming paths and perturbation outcomes. We highlight dynamo’s power to overcome fundamental limitations of conventional splicing-based RNA velocity analyses to enable accurate velocity estimations on a metabolically labeled human hematopoiesis scRNA-seq dataset. Furthermore, differential geometry analyses reveal mechanisms driving early megakaryocyte appearance and elucidate asymmetrical regulation within the PU.1-GATA1 circuit. Leveraging the least-action-path method, dynamo accurately predicts drivers of numerous hematopoietic transitions. Finally, in silico perturbations predict cell-fate diversions induced by gene perturbations. Dynamo, thus, represents an important step in advancing quantitative and predictive theories of cell-state transitions.
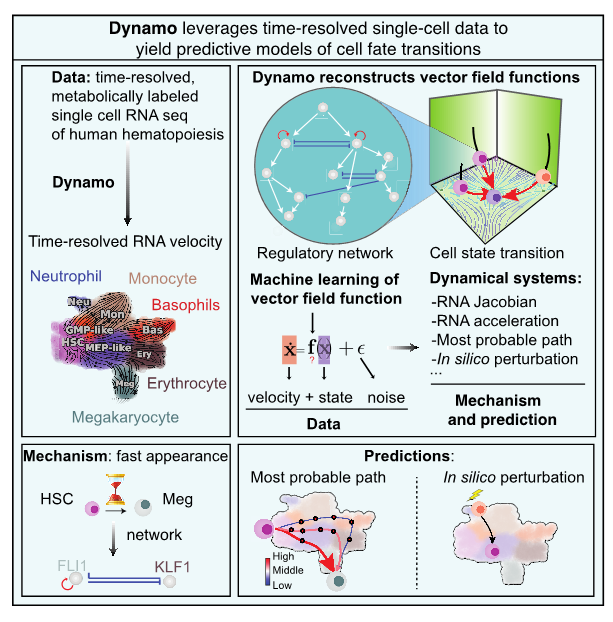
Figure 1. Overview of dynamo computational framework for analyzing time-resolved single-cell data.
Upper left: Dynamo generates time-resolved RNA velocity from metabolically labeled single cell RNA-seq data based on a biophysical model of gene expression.
Upper right: The transcriptomic vector field reconstructed from discrete RNA velocity samples using a machine learning method encodes essential gene-gene interaction information in the underlying gene regulatory network, which can be extracted from various differential geometrical quantities of the vector field.
Lower left: Dynamo reveals the underlying biological mechanism for the fast appearance of Megakaryocytes in a hematopoiesis dataset.
Lower right: Dynamo predicts cell type transitions by mapping out the most probable path and cell fate changes under in silico perturbations.
Whitehead Institute News Article
UPMC News
Qiu X, Zhang Y, Martin-Rufino JD, Weng C, Hosseinzadeh S, Yang D, Pogson AN, Hein MY, Min KH, Wang L, Grody EI, Shurtleff MJ, Yuan R, Xu S, Ma Y, Replogle JM. Lander ES, Darmanis S, Bahar I, Sankaran VG, Xing J, Weissman JS (2022) Mapping transcriptomic vector fields of single cells. Cell, in press.